Dmitry Petrov gives a sneak peek into the recent developments at Iterative.ai and highlights the most exciting trends in AITech right now.
1. Could you tell us more about Iterative.ai’s and what was the inspiration behind starting this company?
As a data scientist at Microsoft, I saw first hand the problems that occur when the data science and software engineering environments don’t work together. There is essentially a wall between the two worlds. I wanted to build a company that would make tools to remove this wall so that the machine learning world and software engineering world could live together and be more productive. This way, machine learning models that are built can be easily put into production apps and services.
2. Could you elaborate on your entrepreneurial journey?
I knew I always wanted to build a company. My journey from a remote area of Russia, through my computer science education, move to the States with a Data Science position at Microsoft, boot-strapping a start-up and becoming and American citizen has been long, but so rewarding. I’m proud of the products we are building and the team that is building them.
3. Can you tell us about the products and services that Iterative.ai offers?
Iterative’s mission is to deliver the best developer experience for machine learning teams by creating an ecosystem of open, modular ML tools. This mission was born out of the need for harmoniously joining the best practices of software engineering with machine learning projects that are driving a growing number of software applications today. DVC (Data Version Control), the first tool of the suite, extends the versioning power of Git to the large and often unstructured datasets used in ML projects. For the first time data, pipeline and experiments could be versioned reliably and reproducibly, supporting the data-centric movement and the growing need for data governance in ML projects.
CML (Continuous Machine Learning), the next tool in Iterative’s lineup, provides CI/CD capability to ML projects. This helps teams automate and orchestrate resources, whether in the cloud or on prem. The addition of TPI (Terraform Provider – Iterative) extends this functionality further, enabling the management of spot instances in multiple clouds to conserve resources when running large models.
MLEM, our latest tool, simplifies ML model deployment and is the basis of a full model registry, something many companies are working on in their ML teams.
We know that the one size fits all monolith platform will not work for all ML/AI teams. ML teams need flexible tooling that enables them to manifest their visions for their ML projects without the heavy lift of building that tooling from scratch. Our aim is to create these tools for them to allow them to iterate fast, use compute and people resources efficiently, enable cross-team collaboration, and provide as frictionless as possible deployment tool so that they can focus on solving their domain problems. We are building the tools to accomplish this task.
4. What makes your offerings unique compared to the competitors?
Many competitors are building SaaS solutions that might satisfy the needs of data analysts and some data scientists, but ML engineers and researchers need an environment that is closer to a software development environment. ML engineers need: command line, Git, CI/CD, IDE such as VScode etc. This happens because ML engineers spend the majority of their time coding and we meet them where they are.
All of our tools are GitOps-based, matching with software teams using Git as the source of truth for all applications. Information and versioning around ML models and experiments, data, artifacts, and hyperparameters, are all stored within an organization’s Git service. This GitOps approach aligns the ML model development lifecycle with that of an organization’s apps and services so they get faster time-to-market with transparent collaboration between software development and ML teams.
5. What are the pain points that customers approach you with?
As the machine learning and modeling space is in a nascent stage, there aren’t established best practices or MLOps tech stacks for data scientists and organizations in general to emulate.
A lot of the tools on the market today aren’t built with data scientists in mind – they focus more on business users.With this approach, the developer experience isn’t the best and models under development are hampered and have a slower time-to-market.
6. What excites you most about your industry?
The possibilities. There are so many problems that can be solved in this world with responsible ML/AI. The goal is to figure a way to reduce the tooling burden on teams so they can focus on the issues at hand in their domains.
7. Could you give a sneak peek into the recent developments at Iterative.ai?
We’ll be continuing to develop new tools for ML engineers and data scientists, with a couple new ones being released later this year. On the commercial side, we’ll also be continuing to add on enterprise-grade features to meet the demands of AI- and ML-first organizations that use our tools. Our tools are meant for collaboration and we’ll continue to develop solutions with this focus. Other plans will include additional security controls as well as features around data discovery/management and further development on our existing tool set. We’re the first to offer a data catalog around unstructured data for machine learning use cases and will continue to innovate around this as the AI and ML market matures in the next 5 years.
8. Let us in on Iterative.ai’s work culture. How would you describe your team?
We are a group of passionate, pioneering people that are willing to roll up our sleeves and tackle the hard questions, take calculated risks, and deliver work we can be proud of. As our all-remote team has grown, we have developed and continue to focus on developing the internal practices needed to build strong relationships amongst our team members across the world. Our team is building strong bonds in the process
9. In your opinion, what are the most exciting trends in AITech right now?
We predict a shift to a developer-first experience in ML. More integration of ML tools to development environments such as IDE, version control as well as GitOps based ML model deployment systems. ML engineers will embrace more and more software development best practices.
Data centric AI is a big trend in ML model development. We expect that some of these solutions will be democratized and released as widely available open source tools.
10. What are your plans for expansion and growth? How do you see the company and the industry in the upcoming years?
We’ll continue to work on our open-source tools and supporting the community around them as that is where the company started from. On the commercial side, we’ve been seeing strong demand around our solution for larger ML teams that need to collaborate as they get their models to market. We’ll invest actively in product development, sales, and marketing to support the demand.
In the coming years, we see the industry and ML teams use a large mix of machine learning tools. This will reflect back to our products where we’ll focus on making open, modular tools so that data scientists and ML engineers can continue to use the tools they want as they add in various MLOps solutions into their workflows.
11. What is the biggest piece of advice you would give to company leaders?
As a remote-first company, constant and even over-communication has been important to our success. As you grow your company, I would emphasize transparent, frequent and repetitive communication across all channels – Slack, email, all-hands meetings, etc. so all team members know what’s going on and how what they’re working on contributes to the company. This fosters a better environment for team members to know exactly how they’re impacting the company and how the company is doing.
12. Where do your passions lie? What do you think defines you as a person?
I built Iterative around our open-source tool, DVC, which helps data scientists and ML engineers version data, experiments, and pipelines. Iterative combines my interests around data science and entrepreneurship into a single project. I’ve enjoyed supporting the open-source community that I’ve built and continue to build – many data scientists are doing important and innovative work and it’s gratifying to know that they use many of our tools to do so.
13. Which motivational quote drives you to achieve more at work?
“Far better it is to dare mighty things, to win glorious triumphs, even though checkered by failure, than to take rank with those poor spirits who neither enjoy much, nor suffer much, because they live in the gray twilight that know neither victory nor defeat.” – Teddy Roosevelt
Visit AITechPark for cutting-edge Tech Trends around AI, ML, Cybersecurity, along with AITech News, and timely updates from industry professionals!
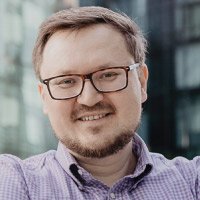
Dmitry Petrov,
Co-Founder & CEO at Iterative
Dmitry Petrov is the co-founder and CEO of Iterative. He served previously as a Data Scientist at Microsoft and has a PhD in Computer Science.