An interview with JAD Bio CEO and Co-founder Ioannis Tsamardinos about analyzing customer behavior
1. Tell us how you came to be the CEO at JADBio. How much of your typical day is involved in innovating AI tech for your customers?
I was working on automated machine learning for bioinformatics for a while as an academic. But, I always had the desire to commercialize our ideas and technology to create a system that consolidates all of our research into one, practical platform; a product that turns esoteric research to commonplace applied technology. The 2008-2009 economic crisis gave me the push that I needed to decide to transition to being an entrepreneur. We started with contractual services to bootstrap funding for JADBio’s development. But the turning point came in the summer of 2018 when I reconnected with an old Danish associate of mine. He liked the prototype of JADBio I showed to him. He had access to investors and believed in its potential. A year later JADBio received its first investment and landed its first big client.
My typical day is split between organizational, administrative, and legal aspects of running the company and developing new AI tech for our customers. The latter is of course the more fun part of it. There is a load of new tech and ideas that is in the prototype stage and we are eager to put into production. I cannot wait to see them in action and get feedback from our clients.
2. What are the applications or rather opportunities you seek to have with your product?
We want to bring machine learning to anyone in biomedicine. Some big life science industries are using our product, but I would like to see it applied more in Big Pharma.In addition, I think JADBio could be a game-changer in the teaching, application, and research of molecular biology and so we are now giving webinars to US universities to get the attention of students, post-docs, and researchers.
3. How did you define the vision of JADBio? How did you approach your first 100 days as the CEO at JADBio?
The first 100 days at JADBio I think can be described as an all out-of-control but productive chaos. We tried to hire, clarify strategy, objectives, KPIs, roles, responsibilities, budgets and build the foundation for scaling up. At the same time, we were working feverishly on releasing the product, testing, and getting feedback, while all the while balancing this with our service responsibilities and existing contracts. You throw a bunch of balls in the air and try to juggle.
4. What are some of the unique lessons you have learnt from analysing your customer behaviour?
Well, one lesson is that no detail is too small. It may be a feature or functionality that seems trivial but it could make a world of difference to a client. The other is that in a startup you have more needs than people, money, or time. So, it’s very important to prioritize and have the procedures in place to do so. That means that some clients have to wait longer than desired, but there is no other way. Finally, human contact with clients is important and one has to balance between massive adoption and automated sales on the net with personally contacting customers.
5. What are some of the distinctive features of JADBio’s platform? And how do you differentiate yourself from your competitors?
JADBio has numerous distinctive features and competitive advantages as an Automated Machine Learning tool (AutoML). We don’t just put existing algorithms together; we innovate. JADBio has some breakthrough algorithms that solve open problems in machine learning.
First of all, JADBio is fully automated. Anybody can use it and perform a sophisticated and correct machine learning analysis even if they do not know any math, statistics, or coding. All they need is an Excel file with their data.
Second, even though JADBio is a general AutoML tool, it is the only AutoML platform specifically designed for life science data and particularly molecular data. This means that it can deal with the idiosyncrasies of molecular data such as very low sample size and very high number of measured quantities that could reach to millions. More importantly, it deals with common analysis needs of life scientists. A biologist may not need a predictive model for predictions! They may need it to understand what are the features and biomarkers that are predictive and important, what is their role, and get intuition about the molecular mechanisms involved. Knowledge discovery is often more important than a predictive model. So, JADBio focuses on feature selection and its interpretation.
Third, JADBio doesn’t overestimate performance! You can trust the estimation of how predictive your model is. The implications are crucial. You do not need to hold out a separate chunk of your data for validation and “lose samples to estimation” as we say in statistics. In contrast, our computational experiments confirm that many competitors systematically overestimate performance! So, you need to lose part of your data to validate the model and that is sometimes unacceptable: you cannot afford to wait until thousands of people have died from Covid-19 to validate your model. You need to develop a model, estimate its performance, and use it right here, right now with as few subjects as possible.
6. Indivumed recently launched Groundbreaking AI Platform, with the AutoML based on JADBIO technology to Leverage Multi-omics Data for Discoveries in Oncology. Can you elaborate more on the same?
Invidumed is a strategic partner, investor, and the first big client for JADBio. They have incorporated JADBio fully into their biomedical analyses. They have now launched a new AI platform for oncology that combines the unique measuring quality and richness of their multi-omics oncology data, with oncology-specific AI functionalities, and the power of JADBio. We are very excited about the possibilities and prospects and in anticipation of new discoveries in oncology.
We designed JADBio with an architecture that makes it easy to use as embedded software within other platforms, adding AutoML capabilities to third-party software. Invidumed is embedding JADBio within their platform, but JADBio could be embedded into any other software, from Life science AI to cars and laptops to make them learn and adapt to users’ behavioral patterns.
7. JADBio was recently in the news for raising €800,000. How are you planning to utilise it?
We recently raised € 800,000 euro, and we have received several EU grants to supplement our cash reserves. We now focus on business development, operations, marketing, privacy and security, and scaling up the company. We opened subsidiaries in Denmark and the US. Our target and first priority now is growth. We want everybody to know they can use JADBio and analyze their data, even if they are not expert analysts.
8. What advice would you like to give to the upcoming AI-based Data Analytics’ start-ups?
Competition is fierce and there is too much hype. Everybody is claiming they are doing AI, machine learning, and data analytics. It’s hard to separate the “noise from the signal” as engineers say, i.e., truly innovative and true AI products from products that just happened to use the buzzword du jour as a marketing ploy. So, there has to be clarity in terms of message, positioning in the market, and uniqueness of the offering.
9. Can you give us a sneak peek into some of the upcoming product upgrades that your customers can look forward to?
My pleasure. We have algorithms in prototype stage for feature selection and modeling with datasets measuring tens of millions of features and practically infinite sample size, or as much as you can store on the disk. This means JADBio will be able to handle genetic datasets measuring millions of genetic markers, multi-omics data, and combinations of clinical, lifestyle and anything else you can measure. We have AutoML for images, signals, and free text even when sample size is small, all with one click. Other useful functionalities, coming up, have to do with decision support with the use of the model, explainability of the model, and interpretation. Also, functionalities that allow the batch analyses of hundreds of datasets with one click. So, stay tuned!
10. Which is the one AI breakthrough you will be on the lookout for in the upcoming year?
I am hoping for breakthroughs in the automation of Causal Discovery. Right now there is theory and algorithms for that, but it is an esoteric subject confined to a few academics. We are working on automating it, let’s call it AutoCD, and making accessible to everyone the discovery of causal relations from data. Very soon the industry will realize that predictive analytics are not enough. We don’t only need to know what predicts customer attrition. We need to know what causes it, so we can intervene. In biomedicine, knowing the causal-effect relations means we treat the causes of disease and not the symptoms and design new drugs. We are also working on AutoML with images, free text, and medical signals that could be a game changer for many application domains.
11. Please share a recent piece of content (can be video, podcast, blog, movie, webinar) that resonated the most with you with respect to AI or your work in general?
I was particularly excited to hear about the program Lean by Microsoft Research that helps to verify mathematical proofs, presented in the YouTube video: https://www.youtube.com/watch?v=HL7DEkXV_60&ab_channel=QuantaMagazine . Can you imagine doing engineering, like sending a man on the moon, without calculators? It’s inconceivable (although it did happen, thanks to “human calculators” at NASA). I believe in the near future it will be inconceivable to do pure math without computers. Some math proofs are so big and complicated (the proof of Fermat’s last theorem is about 200 pages) and only a few mathematicians in the world are able to check their correctness. What if these proofs are wrong and we missed something? Which means that we may be doing math based on false proofs! Big chunks of mathematics could crumble. Programs like Lean verify that what mathematicians do is correct and we can base future math on existing results.
12. What is the one leadership motto you live by?
As a leader you have to inspire and lead by example. You have to listen to all, but then be decisive. Good ideas could come from anywhere and different people are experts in different domains. But, then you have to make a decision and commit.
Visit AITechPark for cutting-edge Tech Trends around AI, ML, Cybersecurity, along with AITech News, and timely updates from industry professionals!
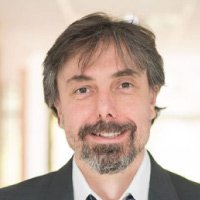
Ioannis Tsamardinos
CEO and Co-founder, JAD Bio
Dr. Ioannis Tsamardinos is a Professor of Artificial Intelligence and CEO at JADBio, the only AutoML platform specifically designed for life science data and particularly molecular data. He has published over 110 scientific papers in international journals and conferences on the development of novel, state-of-the-art algorithms and theory for Machine Learning, Bayesian Network learning, Variable Selection, and Causal Discovery. His work on automatic time-limited actions design and was applied to the NASA Dean Space I software. In his spare time, he is an enthusiastic underwater diver.