Steve Harris from Mindtech talks about the rise of AI Technology and synthetic data solutions
1. Tell us about yourself and your role at Mindtech
After working in the Software and Semiconductor industries for more than twenty years, I and the early founders of Mindtech – friends who I’d known for a long time – started to get curious. Would it be possible, we wondered, to develop and deliver AI applications as a “Neural Network platform” on a chip?
Fascinating though that thought was, we hit some unexpected roadblocks. Working with three lead customers, they each shared a huge amount of real-world visual data for us to train the networks. What we discovered when we looked at the data astounded me.
Firstly, the data across all three customers was very, very poor, and mostly completely unusable. That’s because it was either not the right data, or it was not in any way privacy compliant, with no data provenance. A big red flag.
Secondly, the small amount of data that was usable took an inordinate amount of time to properly annotate because the process depended on humans. Real humans, painstakingly picking out pixel after pixel, hour after hour, day after day. It just wasn’t scalable.
And finally, when we looked at acquiring more data from bigger platforms, we got a hard no. Those platforms, if they had good data, knew the value and there was no way they were going to share it.
At that moment, We realised that before we could take the next big leap in AI, we first needed to fix the issue of providing training data for AI systems. Real-world visual data was, and still is, a scarce and precious resource. It has an important role to play in training visual AI systems, but we need an additional training data source that’s unlimited, unbiased, precisely annotated and above all privacy compliant.
That’s when the idea behind Mindtech’s Chameleon Platform was born
2. What’s the problem Mindtech solves?
Mindtech Global is the developer of the world’s leading end-to-end ‘synthetic’ data creation platform for the training of AI vision systems.
Right now, if you’re an engineer or data scientist working on a visual AI system, you’ll be spending around 80% of your time on gathering, cleaning, and manually annotating real-world imagesNot only are these images a scarce resource, but annotating a few frames takes hours. Up to 20 weeks just to get 100,000 high-quality annotated images to start training a visual AI system is a long time, and a challenge we think synthetic data can solve.
3. Mindtech recently announced a successful pre-series A funding round. Can you tell us a little more about that?
We’ve just closed a $3.25m (£2.35m) investment from Mercia, Deeptech Labs, and In-Q-Tel. The round was led by NPIF – Mercia Equity Finance, which is managed by Mercia and is part of the Northern Powerhouse Investment Fund (NPIF)*, with Deeptech Labs and In-Q-Tel participating. The investment will enable the company to accelerate product development at its new engineering base in Sheffield, UK, and support its growing customer engagements across Asia, Europe, and the US.
4. You also just released an update to your AI platform, tell us about that.
The company’s Chameleon platform is a step change in the way AI vision systems are trained, helping computers understand and predict human interactions in applications ranging across retail, smart home, healthcare, and smart city.
We launched Chameleon in 2019 to help companies developing visual AI systems create photo-realistic 3D worlds and extract unlimited synthetic data to train their AI to understand and predict the way humans interact both with each other and the world around them.
This latest platform update brings an enhanced user experience because it simplifies the creation of complex scenarios with comprehensive new data discovery, bias, and diversity management tools. New automated real-world behavioral models built into the simulator ensure the required training datasets are built with ease.
For the first time, data scientists, and machine learning engineers can directly create the precise annotated images they need to train their visual AI systems, with semi-automated tools enabling the rapid evolution of training datasets whilst working within their existing MLOps workflows.
Chameleon’s end-to-end approach saves customers significant time and cost over traditional image sourcing, annotation, and management.
Some of the new and enhanced features include:
- Scenario builder: allowing users to rapidly create application-specific scenarios and corner-case images.
- Simulator: AI driven, automated real-life behavior modeling to create datasets that mimic the real world in both look and statistical attributes. Plain-text control scripts allow engineers to use a seamless workflow between CLI and UI based tools.
- Curation Manager: Visual analysis of synthetic and real datasets, identifying diversity and bias.
- The new Domain Randomization pack: allowing users to abstract events under observation from the background and other interactions, permitting rapid creation of the structured and unstructured images required for training robust, accurate networks.
5. So, what’s next for the company?
We’re hoping we see more companies come to view synthetic data as a really powerful tool for AI and business growth— especially when the evidence is there to support this already. A 2020 report by McKinsey noted that 50% of high-performing AI companies already use synthetic data to train AI models when there is insufficient real-world data.
Likewise, Deloitte Consulting found that using an AI model trained with 80% synthetic data had similar accuracy to a model trained on all real data. These results just show the direction we’re heading in. By 2024, Gartner predicts that 60% of data used for AI and data analytics projects will be synthetic, and by 2030, synthetic data will have completely overtaken real data in model training.
We’re seeing encouraging use cases already: machines are being trained to monitor patients recovering from surgery; security and surveillance systems are being trained to detect suspicious objects or unusual patterns of behaviour, such as lost children, inside densely populated public spaces; and service robots are being trained to understand social distancing in the world full of humans.
We’re moving on rapidly from those big red flags we spotted in the early days of Mindtech; I can’t wait to see how far the impact of this synthetic solution can go in the real world.
You can find out more about how synthetic data is created and applied with our Mindtech video showcase here.
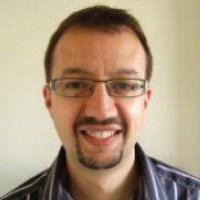
Steve Harris
CEO, Mindtech
Steve is CEO of Mindtech with over 30 years experience in the technology market sector. Steve has been instrumental in creating several start-up organisations in Europe and brings with him a track record of success in building strategic relationships and strong revenue streams with tier one companies worldwide. Prior to joining Mindtech Steve held senior positions in sales and business development in leading technology companies including Imagination Technologies, Gemstar, Liberate and Sun Microsystems. Steve holds a masters in Microprocessor Engineering from Manchester University.